Quick Summary:
Financial institutions today face growing pressure to serve customers faster, stay compliant, and operate more efficiently all while managing tighter resources. In this environment, AI Assistants are emerging as a powerful solution. Powered by Generative AI and Natural Language Processing (NLP), they go beyond basic chatbots by understanding context, executing tasks across CRM and ERP systems, and delivering personalized, scalable support. For banks and fintech firms alike, these AI-driven tools are streamlining operations, reducing costs, and opening doors to new growth.
In this blog, we’re going to discuss📝
- Key Use Cases of AI Assistants in Financial Services
- Business Benefits of AI Assistants in Financial Institutions
- Tailoring AI Assistants for Small, Mid, and Large Financial Organizations
- Real-World Examples: AI Assistants Driving Transformation in Finance
- Technologies Powering AI Assistants in Finance
- Implementation Plan for AI Assistants in Financial Services
- Cost and Maintenance Considerations for AI Assistants in Finance
- The Future of AI Assistants in Finance
The finance industry is at a crossroads.
On one side: rising customer expectations, operational complexity, and the constant pressure to reduce costs.
On the other: outdated processes, siloed systems, and support teams stretched thin.
So how do financial institutions adapt, without breaking the bank?
Well, they turn to AI Assistants.
Not to be confused with old-school chatbots that follow a rigid script, AI Assistants are powered by Generative AI and Natural Language Processing (NLP). They understand intent, hold contextual conversations, and even perform tasks like pulling data from a CRM or helping users complete a loan application.
Here’s what sets AI Assistants apart:
- They understand natural language, not just keywords.
- They connect with internal systems like CRMs and ERPs to take action.
- They’re always available across channels, around the clock.
- They improve over time with machine learning and feedback.
More than a tech upgrade, AI Assistants offer real business value.
- Automate routine queries and reduce support volume.
- Offer personalized financial guidance without human delays.
- Cut processing costs in areas like loan origination or retirement planning.
- Scale service without scaling the team.
Whether you’re a small credit union or a global bank, the shift is already happening.
Key Use Cases of AI Assistants in Financial Services
AI assistants are no longer just tools that answer simple questions. They’ve evolved into strategic enablers across the entire financial services landscape. Whether serving a customer at 11 PM or helping an employee locate underwriting criteria in seconds, these assistants are transforming how financial institutions operate in both situation externally and internally.
Let’s explore some of the most impactful use cases where AI assistants bring real value:
Customer Support That Never Clocks Out
Today’s customers expect answers on their schedule, not yours. AI-powered financial chatbots provide 24/7 self-service support, resolving routine inquiries like account balances, card limits, or transaction history instantly.
With multilingual capabilities, customers can interact in their preferred language, breaking down communication barriers and enhancing satisfaction across diverse demographics.
Example:
User: “Can I check my credit card bill in Spanish?”
AI Assistant: “Claro. Aquí está tu último resumen: $325.20. ¿Deseas ver el detalle?”
Smarter Loan Origination Without the Paper Chase
Applying for a loan often means endless back-and-forth and document uploads. AI assistants simplify this by guiding users step-by-step through the loan process from eligibility checks to document collection and final submission.
They can pre-fill known information, validate entries in real time, and escalate cases when something looks off. This reduces drop-offs and speeds up turnaround times.
Imagine a user saying, “I want to apply for a home loan,” and the assistant instantly responds with:
“Great. Based on your profile, you may be eligible for up to $250,000. Let’s start with your income documents.”
The entire workflow becomes faster, smarter, and more personalized exactly what today’s borrowers expect.
Personalized Investment & Retirement Planning
No one wants a generic financial plan. AI assistants analyze a user’s transaction history, goals, and risk appetite to offer customized retirement and investment suggestions.
Think of it as having a virtual financial planner who’s always available, can explain things in plain language, and evolves with your needs.
“You saved 22% more this quarter than last. Want to explore short-term investment options?”
That’s proactive, contextual guidance powered by NLP and real-time data.
For wealth management firms and advisory services, these assistants improve client retention while adding a layer of automation to the advisory process.
Internal Agent Support & Knowledge Access
Financial institutions don’t just serve customers they support internal staff too. AI assistants help employees search policies, fetch rates, summarize procedures, or even draft responses saving minutes on every task.
Instead of agents digging through outdated wikis or spreadsheets, they can just ask:
“What’s the underwriting guideline for high-risk auto policies?”
…and get an accurate, up-to-date answer instantly.
This kind of internal enablement boosts productivity, reduces onboarding time for new hires, and ensures compliance with evolving procedures.
CRM/ERP Integration for Seamless Task Execution
The real power of AI assistants in finance unfolds when they integrate with core systems like CRMs and ERPs. By syncing with customer records, transaction histories, and task management tools, assistants can initiate actions not just answer questions.
- Update customer contact info
- Trigger a follow-up call task in the CRM
- Log a service request or payment reminder
- Pull real-time financial summaries for advisors
Financial Literacy & Customer Education
AI assistants can proactively educate users about financial concepts, helping them make better decisions.
- Explain terms like APR, compound interest, or credit utilization
- Offer tips on managing debt or saving for retirement
- Provide contextual advice based on the user’s financial behavior
This builds trust, improves digital engagement, and can be particularly useful for Gen Z and underbanked populations.
Business Benefits of AI Assistants in Financial Institutions
In the financial sector, technology isn’t just about efficiency but it’s about building trust, improving service, and staying agile in a fast-changing environment. AI Assistants bring all of that to the table.
But to truly understand their impact, it helps to look at what they enable and how they do it in real-world scenarios.
Context-Aware Customer Support at Scale
In finance, timing and clarity make all the difference. Customers often reach out with urgent questions whether it’s about a pending loan, payment delay, or investment query. Waiting on hold or browsing through outdated FAQs doesn’t cut it anymore.
AI Assistants bridge this gap by being:
- Available 24/7
- Context-aware
- Capable of tailoring responses based on CRM data
Customer: “Can I check if I’m eligible for a home loan?”
AI Assistant: “Sure. May I know your monthly income and current employment status?”
→ The assistant instantly checks loan eligibility logic, logs the interest, and routes the customer to a human advisor.
Business Impact:
- Increases lead capture by responding instantly
- Reduces drop-off rates during the inquiry stage
- Keeps the customer engaged even during off-hours
- Builds trust with a smooth, responsive experience
Operational Efficiency Through Automation
Support desks in banks and financial firms are overwhelmed and not because of complexity, but because of volume. Dozens of staff handle hundreds of repetitive tasks: checking balances, issuing account statements, routing service requests.
AI Assistants can offload these routine tasks in real time without compromising accuracy.
Employee: “Can you get me the latest interest rates for education loans?”
AI Assistant: “Sure. For students under 25, the current rate is 7.1%. Would you like this in a formatted PDF?”
Business Impact:
- Reduces internal support workload
- Minimizes time spent on low-value tasks
- Cuts operational costs tied to customer service
- Ensures consistency in responses across branches
And the best part? It scales. Whether you have 10 requests or 10,000, the AI handles them with the same speed and accuracy.
Revenue Growth from Smarter Interactions
Traditional upselling often feels forced or generic but not when it’s guided by real-time context. AI Assistants understand customer preferences, financial behavior, and past interactions. That makes their recommendations timely and relevant, not salesy.
Customer: “I’m planning a trip. Can I increase my card’s credit limit?”
AI Assistant: “Absolutely. Based on your history, you’re pre-approved for a $2,000 increase. Would you like to activate that now?”
Business Impact:
- Boosts product uptake through natural recommendations
- Increases wallet share per customer
- Empowers sales teams with qualified leads
- Enhances lifetime customer value
Built-In Compliance and Audit Readiness
In regulated industries like finance, consistency isn’t a nice-to-have but it’s a necessity. Misstatements, missed disclosures, or forgotten legal disclaimers can lead to fines or worse.
AI Assistants are programmed to follow compliance protocols, document every interaction, and ensure nothing important gets skipped.
Customer: “Can I withdraw funds from my retirement account early?”
AI Assistant: “According to federal guidelines, early withdrawal before age 59½ may result in a 10% penalty. Would you like to view the official document?”
Business Impact:
- Ensures every customer gets the correct, compliant answer
- Logs every step for audit trails
- Minimizes regulatory risk
- Reduces training overhead for support staff
And since every conversation is logged, your compliance team doesn’t need to chase records, everything’s already in place.
Real-Time Analytics and Intent-Based Insights
Every conversation holds a clue. AI Assistants don’t just respond but they learn. Over time, they identify:
- Which services are most requested
- What friction points delay conversion
- Where user confusion typically happens
🗨️ Customer: “What’s the best account type for freelancers?”
🗨️ → The AI Assistant answers, logs the question, and it becomes part of a pattern showing rising demand for self-employed banking solutions.
Business Impact:
- Reveals new product opportunities
- Highlights content gaps or policy issues
- Helps CX, product, and marketing teams make better decisions
- Supports continuous optimization of service journeys
Tailoring AI Assistants for Small, Mid, and Large Financial Organizations
One of the most underrated strengths of a modern AI Assistant in finance is its scalability.
Unlike legacy systems that require heavy lifting to implement and maintain, AI Assistants can be right-sized adapting to the needs, constraints, and ambitions of different financial institutions. Whether you’re a lean fintech startup or a complex enterprise bank, the assistant grows with you and not against you.
Small Financial Firms: Big Impact Without Big Overhead
Smaller institutions like local credit unions or niche investment advisors often have limited staff and even tighter tech budgets. But they still face the same customer demands as large banks: fast responses, clear information, and reliable service.
That’s where lightweight, plug-and-play AI Assistants come in.
- Handle FAQs, appointment scheduling, and basic loan inquiries
- Integrate with simple CRM tools like HubSpot or Zoho
- Offer 24/7 availability without hiring round-the-clock staff
Customer: “What documents do I need for a personal loan?”
AI Assistant: “You’ll need a valid ID, proof of income, and your credit report. Want me to send the checklist to your email?”
Why it works:
These assistants require minimal IT setup, are affordable to run, and deliver a professional digital experience that builds trust especially important for growing customer bases.
Mid-Sized Institutions: Scaling with Smarter Workflows
Mid-sized banks and financial service providers sit in a tricky spot but they’re often too big for manual workflows but not big enough to afford massive automation platforms.
AI Assistants help bridge that gap by:
- Automating onboarding processes
- Managing multi-step loan applications
- Syncing with CRMs and basic ERPs for smoother service delivery
- Supporting hybrid workflows with human handoffs when needed
Customer: “Can I finish my mortgage application later?”
AI Assistant: “Sure. I’ve saved your progress. You can resume anytime using your email link. Would you like a reminder tomorrow?”
Why it works:
They reduce support costs, accelerate sales cycles, and create consistent customer journeys without demanding a full-time AI team to maintain them.
Large Enterprises & Banks: Orchestrating Complex, Multi-Channel Operations
Enterprise-level financial institutions operate across regions, products, and compliance frameworks. Their needs go beyond basic automation as they require intelligence, adaptability, and control.
Here, AI Assistants evolve into domain-specific AI agents and they are deployed across different departments like retail banking, wealth management, risk, and compliance.
- Deep integration with enterprise CRMs like Salesforce
- Real-time data retrieval and analytics across channels
- Automated handling of policy checks, KYC workflows, and escalation rules
Customer: “I need help understanding my investment performance this quarter.”
AI Assistant: “Pulling your portfolio summary… your growth has been 6.3% this quarter, driven by gains in emerging markets. Would you like a detailed breakdown?”
Why it works:
Enterprise-grade AI Assistants act as intelligent layers on top of fragmented systems unifying data, supporting advisors, and ensuring every customer gets an informed, personalized response – at scale.
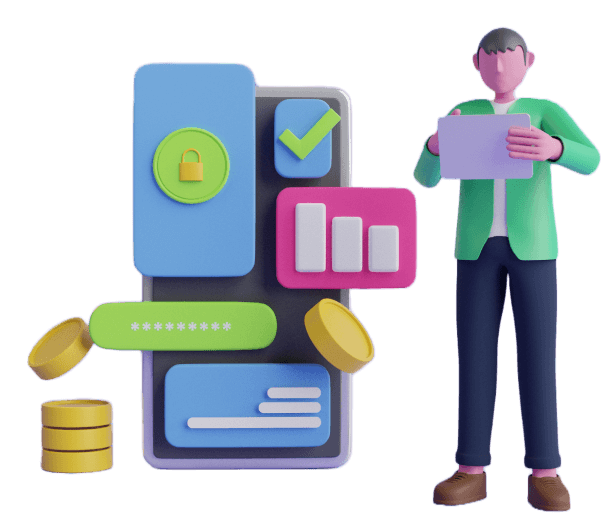
Looking for BFSI IT Solutions?
Get fast-track BFSI digital transformation with secure, scalable solutions!
Real-World Examples: AI Assistants Driving Transformation in Finance
The adoption of AI Assistants in finance is not just theoretical; numerous institutions have successfully implemented these technologies to address specific challenges and achieve measurable outcomes.
Goldman Sachs: Enhancing Internal Operations with AI Assistants
Goldman Sachs has integrated AI tools across its operations, aiming for comprehensive adoption within three years. Key implementations include:
- GS AI Assistant: A ChatGPT-like interface tailored for internal data, facilitating efficient information retrieval.
- Banker Copilot: Assists in drafting documents and analyzing filings, streamlining bankers’ workflows.
- Legend AI Query: Enables natural language searches through extensive data repositories.
These tools have already been deployed to 10,000 employees, with reported efficiency gains of up to 20% among software engineers.
Aviva Investors: Streamlining Portfolio Construction with AI
Aviva Investors, managing £238 billion in assets, has developed an internal AI tool named OptiFI to assist its fixed income team in portfolio construction. This AI Assistant significantly reduces manual workloads, allowing analysts to focus on strategic decision-making. Processes that previously took hours can now be completed in minutes, enhancing overall efficiency.
Morgan Stanley: Automating Administrative Tasks for Wealth Advisors
Morgan Stanley has introduced an AI Assistant named Debrief to automate the drafting of emails and meeting summaries for its 15,000 wealth advisors. This initiative aims to save substantial time by handling manual note-taking, enabling advisors to concentrate more on client engagement and business development. The AI Assistant is expected to save approximately 30 minutes per meeting, representing a significant productivity boost.
Colonial First State: Enhancing Financial Planning with AI
Colonial First State, a major superannuation fund manager, has partnered with Microsoft to deploy an AI tool named Copilot to 75% of its workforce. This AI Assistant aids financial planners by quickly processing complex information related to tax and superannuation legislation, significantly reducing the time needed to find answers. Pilot participants reported saving over 30 minutes daily, indicating a notable increase in productivity.
JP Morgan: Automating Contract Analysis with COiN
JP Morgan has implemented an AI platform called COiN (Contract Intelligence) to automate the analysis of legal documents. This AI Assistant can interpret business credit agreements, reducing the time spent on such tasks from 360,000 hours annually to mere seconds. By automating this process, JP Morgan has significantly improved efficiency and reduced the potential for human error.
Technologies Powering AI Assistants in Finance
Let’s take a look behind the scenes.
AI Assistants might seem simple on the surface like answering questions, helping with forms, or showing account details but they’re built on a powerful mix of smart technologies working together.
Here’s what powers them.
Generative AI and LLMs: Making Responses Feel Human
At the core of modern AI Assistants are Large Language Models (LLMs) the same technology behind tools like GPT. These models are trained on vast amounts of language data, which allows the assistant to generate responses that sound natural, relevant, and helpful.
In practice, this means:
- Tailoring answers to each customer’s context
- Summarizing complex policies in plain language
- Responding dynamically instead of relying on fixed scripts
For example:
🗨️ Customer: “Can I use my credit card internationally?”
🗨️ AI Assistant: “Yes, your card works in over 180 countries. A 1.5% international transaction fee applies. Would you like tips to avoid extra charges?”
Natural Language Processing (NLP): For Understanding What People Really Say
Before the assistant can answer, it has to understand the question that even if it’s asked in a casual or messy way. That’s where NLP comes in.
It helps the assistant:
- Understand common language, even with spelling errors
- Recognize the meaning behind the words
- Sense urgency or emotion
💬 Customer: “My loan is stuck again 😡”
💬 → The assistant understands the tone, treats it as urgent, and offers quick help
CRM and ERP Integration: For Taking Action
AI Assistants don’t just talk but they can also do things. To do that, they connect with tools your team already uses, like your CRM (Customer Relationship Management) or ERP (Enterprise Resource Planning) system.
This allows the assistant to:
- Check balances or loan details
- Update customer information
- Log tickets or schedule calls
💬 Customer: “I moved recently. Can I update my address?”
💬 AI Assistant: “Sure. Your new address is now updated. You’ll get a confirmation email shortly.”
Architecture Considerations for Scalable AI Assistant Deployment
Building an effective AI Assistant in finance goes beyond model selection, it requires a well-structured architecture that supports scale, integration, and adaptability.
Key components to consider:
- Modular Design: Separate layers for NLP, business logic, and integrations ensure flexibility across use cases and departments.
- API-Driven Communication: Enables seamless data exchange between the assistant and systems like CRM, ERP, or core banking platforms.
- Containerization and Orchestration: Using Docker/Kubernetes allows easier scaling, updates, and deployment in cloud or hybrid environments.
- Centralized Intent and Knowledge Management: Ensures consistency across channels and helps track what your assistant knows and what it needs to learn.
- Security by Design: Embed encryption, role-based access, and audit logs from day one, especially when handling customer financial data.
🗨️ Customer: “Can I get a performance report for my retirement fund?”
🧠 The assistant checks customer identity via CRM, pulls investment data via ERP APIs, and formats a personalized summary using NLP response templates.
🗨️ AI Assistant: “Sure. Your retirement portfolio grew by 5.3% this quarter, mainly due to gains in energy and tech sectors. Would you like a PDF report sent to your email?”
Security and Compliance: Built for Finance
In finance, security is serious.
An AI Assistant must protect customer data and follow rules. That’s why it comes with features like:
- Authentication: To verify user identity
- Logging: To keep a safe record of what was said
- Data privacy settings: To make sure nothing leaks or gets exposed
💬 Customer: “Can you tell me my loan balance?”
💬 AI Assistant: “Sure. Please enter the last 4 digits of your phone number so I can verify your identity.”
Implementation Plan for AI Assistants in Financial Services
Deploying an AI Assistant in a financial organization isn’t about building from scratch, it’s about connecting the right systems, training with the right data, and starting with the right scope. Below is a step-by-step framework designed to help financial institutions roll out an AI Assistant that delivers immediate and long-term business value.
Identify a High-Impact Use Case
The first step is to define where the AI Assistant will make the most difference. Rather than attempting to automate everything, focus on one narrow, high-volume task where response speed and accuracy are critical.
For example:
- Handling loan inquiries (eligibility, interest rates, document requirements)
- Assisting with credit card queries (limits, usage, international access)
- Automating retirement plan FAQs or onboarding support
Why start here?
- These processes are repetitive, rule-based, and already follow a clear script
- Automating them has an immediate impact on operational load and response time
- It sets you up for early success and measurable ROI
💡 Tip: Use historical support data to identify the top 10 queries your team handles daily that’s your first automation target.
Integrate with CRM, Help Desk, and Core Systems
To be useful, the assistant must do more than reply, it needs to take action.
That means connecting to:
- CRM systems (like Salesforce, HubSpot) for customer profile access
- Help desk platforms (like Zendesk, Freshdesk) to log or escalate issues
- Core banking or ERP systems for real-time data like balances, transactions, or policy status
These integrations allow the assistant to:
- Personalize conversations based on existing customer data
- Update records after each interaction
- Trigger business processes (e.g., schedule appointments, send follow-up emails)
This step typically involves:
- Setting up secure APIs
- Mapping data fields for read/write access
- Creating fallback protocols for error handling
Security and compliance should be prioritized here especially when customer PII or financial data is involved.
Train the AI Assistant Using Real Customer Data
AI models, even those powered by LLMs, need relevant context to perform well in financial environments. This context comes from real business documents and past interactions.
The training phase should include:
- Chat transcripts from live agents, filtered by use case
- FAQs and policy documents to build the assistant’s knowledge base
- Standard operating procedures that show how human teams respond in specific scenarios
Don’t forget to:
- Teach the assistant when not to answer and to escalate
- Set tone and compliance rules (e.g., always include disclaimers in investment advice)
- Include edge cases and exceptions these are where assistants often fail
This step is iterative. AI learning doesn’t stop after initial training. Ongoing updates based on new interactions will improve its accuracy and tone over time.
Deploy Gradually and Optimize Through Feedback
Launching the assistant across all channels and customer segments at once is risky and rarely effective.
A more controlled approach would be:
- Internal pilot: Let staff test it for internal queries and give feedback
- Soft launch: Offer the assistant on your website/app for one department or service line
- Full rollout: Expand across all relevant touchpoints after performance benchmarks are met
During the rollout phase, monitor key performance metrics:
- Containment rate: % of queries resolved without human handoff
- Escalation accuracy: How often the assistant escalates correctly
- Customer satisfaction: Feedback scores, drop-offs, and complaints
- Usage analytics: Which queries are common vs. where users abandon
Use this data to:
- Improve existing flows
- Add missing intents
- Optimize escalation handoffs
Also, establish a feedback loop between your AI team and business users so frontline teams can report issues and suggest improvements quickly.
Cost and Maintenance Considerations for AI Assistants in Finance
Implementing an AI Assistant in a financial organization isn’t just about building the technology it also involves planning for cost, scalability, and long-term maintenance. While initial estimates for a high-quality AI Assistant can range from $15,000 to $75,000+, it’s important to note that costs are not fixed. They depend on multiple variables such as feature complexity, integration scope, data sensitivity, and ongoing support requirements.
Below are the key factors that influence the total cost of ownership:
Factors That Influence AI Assistant Implementation Costs
Factor | Impact on Cost | Details |
Scope of Use Cases | Low to High | More use cases = more flows, logic, and data to manage |
Level of Customization (Flows/UI) | Medium to High | Prebuilt assistants are cheaper; custom logic and branded UI raise costs |
System Integrations (CRM, ERP, Core) | Medium to High | Connecting to more systems increases API dev and testing time |
Language Support (Multilingual) | Medium | Adds NLP complexity and testing effort per language |
Security & Compliance (Finance-grade) | High | Role-based access, encryption, audit trails, and review cycles add cost |
💬These variables are interdependent and may change as your assistant scales or supports new business areas.
Ongoing Maintenance and Optimization Areas
Even after go-live, AI Assistants require monitoring, fine-tuning, and support to remain accurate and compliant. Below is a breakdown of the primary areas where maintenance effort is needed:
Ongoing Maintenance and Operational Cost Drivers
Maintenance Area | Frequency | Impact on Cost | Purpose |
Model Updates & Retraining | Monthly/Quarterly | Medium | Adapts the assistant to new intents, business logic, and tone changes |
Performance Monitoring & Analytics | Weekly/Monthly | Low | Tracks containment rate, query quality, and escalations |
Data Privacy & Compliance Review | Quarterly | Medium | Ensures all assistant behavior complies with current regulations |
Integration Health Monitoring | Ongoing | Medium | Verifies uptime and stability of CRM/ERP/API links |
Feedback Loop & Flow Optimization | Monthly | Medium | Adjusts based on user behavior and corner cases |
Infrastructure Optimization (Cloud) | Ongoing | Medium to High | Adjusts compute/storage usage based on traffic and performance |
LLM Usage Volume (API Calls/Tokens) | Ongoing | Medium to High | Based on the number and complexity of user queries processed via LLM |
How to Estimate ROI from Your AI Assistant?
A well-implemented AI Assistant should not just be a cost center but it should also produce measurable business value through automation, improved service quality, and better resource use.
Here’s a basic formula to estimate Return on Investment (ROI):
ROI (%) = [(Annual Savings + Additional Revenue) – Total Cost] / Total Cost × 100
Where:
- Annual Savings: Cost reduced from fewer support staff hours, improved process efficiency, lower error rates
- Additional Revenue: Upsell/cross-sell conversions, faster loan processing, reduced churn
- Total Cost: Implementation + operational costs over 12 months
The Future of AI Assistants in Finance
While AI Assistants are already making their mark in support and operations, what’s coming next will go far beyond chat and automation. The future lies in autonomy, intelligence, and proactivity where AI doesn’t just assist but actively drives decisions, detects patterns, and supports both customers and internal teams like never before.
Here’s where the next leap is happening:
Autonomous AI Agents for Decision Execution
The next generation of AI in finance won’t wait for input – it will act.
Autonomous AI agents will:
- Proactively monitor customer portfolios, accounts, and transactions
- Detect anomalies, opportunities, or risks
- Trigger workflows like pre-approvals, escalations, or outreach without human prompts
Example:
An AI agent notices a sudden drop in account activity, checks recent transactions, flags it as a retention risk, and sends a personalized re-engagement offer all done autonomously.
This shifts AI from reactive support to automated operations with real-world business impact.
Predictive Personalization at Scale
Today, AI can recommend credit cards or suggest FAQs. But in the future, it will offer deep, predictive personalization and understanding not just what a customer did, but what they’re likely to do next.
AI Assistants will:
- Analyze behavioral, transactional, and contextual data in real-time
- Anticipate financial needs before the customer expresses them
- Tailor offers, content, and even UX based on individual patterns
Example:
A small business customer receives tailored cash flow guidance during seasonal downturns, based on multi-year transaction trends before asking for help.
This is hyper-personalization powered by predictive AI, not just marketing automation.
AI Co-Pilots for Financial Advisors and Internal Teams
Another major leap will be on the internal side like helping human advisors, underwriters, analysts, and operations teams work faster and smarter.
AI Co-Pilots will:
- Summarize client portfolios or regulatory documents in real time
- Surface risk indicators or cross-sell opportunities during calls
- Draft meeting notes or customer outreach messages instantly
Example:
A relationship manager speaks with a client about expanding their business line. The AI Co-Pilot flags relevant credit products, checks recent engagement data, and drafts a follow-up email all before the meeting ends.
This creates superpowered teams not just automated service.
AI Governance, Explainability, and Risk-Aware Models
As AI takes on more responsibility in financial decisions, the need for explainability and governance will grow.
We’ll see:
- AI Assistants that justify their decisions with traceable logic
- Compliance-ready audit trails for every automated action
- Risk models that adapt based on regulatory feedback or customer permissions
Example:
A customer receives a product offer. The assistant can explain: “This offer was recommended based on your income pattern, current savings ratio, and recent interest in short-term investments.”
This kind of transparent, explainable AI will be essential for trust, compliance, and adoption in finance.
Final Thoughts
AI Assistants are already solving real problems for financial institutions from reducing loan processing time to improving customer service accuracy. They’re not just replying to queries; they’re acting on data, supporting decisions, and creating better experiences for both customers and teams.
Firms adopting this early are gaining more than efficiency. They’re earning trust, increasing retention, and making their operations future-ready – one smart interaction at a time.
The best path forward? Start small. Launch where it matters most. Learn, improve, and expand.
And if you’re looking for a partner to help design and implement an AI Assistant that actually delivers, Aglowid can help you get there thoughtfully, securely, and with business value at the core.
Because in finance, timing matters and the time to begin is now.