Quick Summary:
This blog highlights how NLP in healthcare is transforming patient care, improving clinical decision-making, and streamlining operations. It explores key NLP in healthcare use cases, important NLP models, and the challenges of integration. Ideal for healthcare professionals and innovators looking to understand the impact of NLP in healthcare.
In this blog, we’re going to discuss📝
Natural Language Processing in healthcare industry is revolutionizing healthcare operations. From simplifying medical records to helping doctors make better decisions, NLP tools are having a major impact on improving patient care. This blog will explore the different ways NLP is transforming healthcare, some common use cases, healthcare NLP models, and the challenges to overcome for successful integration.
The Impact of NLP in Healthcare System
Healthcare systems generate huge amounts of data daily. Much of this data is in unstructured formats, like doctor’s notes, patient records, and research papers. NLP helps to process and analyze this text data in ways that were once impossible. By applying AI and machine learning algorithms, NLP can understand, interpret, and extract valuable information from vast amounts of medical text.
Stats showcasing impact of NLP in Healthcare Industry
- The global NLP in healthcare and life sciences market was valued at USD 4.9 billion in 2023, with a projected CAGR of 34.7% from 2024 to 2030 as per Grand View Research.
- The global healthcare NLP market was valued at USD 3.19 billion in 2023 and is projected to reach USD 10.73 billion by 2031, growing at a CAGR of 16.67% as per Kings Research.
The Role of NLP in Improving Patient Care
NLP offers several benefits that directly affect patient care and outcomes:
1. Simplifying Healthcare Records
Medical records are often complex and filled with technical jargon. NLP helps convert these records into structured formats that are easier to read and interpret. This makes it simpler for healthcare professionals to access relevant information quickly, improving diagnosis and treatment speed.
2. Matching Patients with Clinical Trials
Clinical trials are critical for medical advancements, but finding the right patients for each trial can be difficult. NLP can help by analyzing patient records and identifying the ones who meet specific trial criteria. This reduces the time and effort required for trial recruitment, speeding up research and potentially saving lives.
3. Helping Doctors’ Decisions
Doctors face countless decisions every day. NLP tools can analyze patient history, clinical notes, and research to provide doctors with evidence-based recommendations. This helps doctors make more informed decisions, potentially leading to better treatment outcomes for patients.
Top 14 NLP in Healthcare Use Cases
How is NLP used in healthcare? Applications of NLP in healthcare are diverse, ranging from improving patient care to streamlining administrative tasks. Here’s an extended look at all the major NLP use cases shaping the industry today:
1. Clinical Trial Management
Clinical trials are essential for advancing medical treatments, but recruiting the right participants can be time-consuming. NLP automates this process by analyzing electronic health records (EHRs) to identify patients who meet specific trial criteria. This can significantly speed up patient recruitment, reduce human error, and ensure that trials are more representative of the patient population.
Key Benefits:
- Faster patient recruitment
- Better match between patients and trials
- Enhanced accuracy in identifying eligible participants
2. Drug Discovery
Drug discovery is a complex, resource-intensive process. NLP tools help researchers analyze vast amounts of medical literature, clinical studies, and patient data. This helps in identifying new potential drug candidates and biomarkers. By scanning medical texts, NLP can uncover patterns that may not be immediately apparent to researchers.
Key Benefits:
- Accelerates drug discovery
- Identifies promising drug candidates more quickly
- Enhances research by uncovering hidden insights
3. Medical Coding and Billing
Medical coding and billing require translating healthcare diagnoses, procedures, and services into standardized codes for insurance and payment purposes. NLP simplifies this process by extracting relevant information from clinical notes and converting it into accurate medical codes. This reduces the manual effort involved, minimizes errors, and ensures faster reimbursement.
Key Benefits:
- Increases billing accuracy
- Reduces coding errors
- Speeds up reimbursement processes
4. Computational Prototyping
In medical research, computational prototyping involves developing predictive models to understand diseases or predict treatment outcomes. NLP plays a vital role by extracting relevant data from medical research papers, clinical notes, and patient records. This information helps build models that can simulate patient responses to treatments and predict health outcomes.
Key Benefits:
- Helps create predictive models for disease and treatment outcomes
- Supports faster R&D in medical treatments
- Enhances precision in clinical decision-making
5. Clinical Documentation Management
Healthcare professionals spend a significant amount of time documenting patient visits, diagnoses, and treatments. NLP can automate the extraction of key details from doctor-patient conversations and written records. Tools such as speech-to-text and automated documentation systems help generate accurate clinical notes without requiring manual input, allowing clinicians to focus more on patient care.
Key Benefits:
- Reduces clinician time spent on documentation
- Improves the accuracy and completeness of clinical records
- Enhances efficiency in healthcare settings
6. EMR (Electronic Medical Record) Implications
EMRs have transformed the healthcare industry, but the vast amount of unstructured data they contain can be challenging to analyze. NLP models help make sense of this unstructured data by extracting valuable insights, identifying patterns, and generating reports. This can improve care coordination, identify gaps in treatment, and support evidence-based decision-making.
Key Benefits:
- Extracts actionable insights from unstructured EMR data
- Improves care coordination and treatment planning
- Enables predictive analytics for better patient outcomes
7. Patient Sentiment Analysis
Patient sentiment analysis involves analyzing feedback from patients, including surveys, social media posts, and online reviews. NLP tools can detect the sentiment behind these texts whether positive, negative, or neutral and provide healthcare providers with actionable insights into patient satisfaction, concerns, and emotional well-being.
Key Benefits:
- Improves patient satisfaction by identifying issues early
- Provides valuable insights into patient concerns and experiences
- Helps healthcare facilities improve services based on real-time feedback
8. Root Cause Analysis
Root cause analysis aims to identify the underlying causes of health problems or systemic issues within healthcare organizations. By analyzing clinical notes, patient outcomes, and treatment histories, NLP can help healthcare professionals identify recurring patterns, misdiagnoses, or treatment failures that may be affecting patient care. This helps prevent future errors and improves patient safety.
Key Benefits:
- Identifies the root causes of patient issues
- Reduces medical errors and improves safety
- Supports quality improvement efforts in healthcare facilities
9. Clinical Entity Resolver
In healthcare, identifying and linking clinical entities (e.g., symptoms, diseases, medications) within text is crucial for accurate information retrieval. NLP models that resolve clinical entities can connect disparate terms to provide a unified understanding. For example, a reference to “heart disease” in one document can be linked to its clinical synonym “coronary artery disease,” making it easier to find related records or research.
Key Benefits:
- Ensures accurate linking of clinical terms and entities
- Improves information retrieval in clinical records
- Reduces errors in patient diagnoses and treatment plans
10. Automated Patient Instruction Generation
Providing clear, accurate instructions to patients is vital, particularly after surgeries or medical procedures. NLP tools can generate personalized patient instructions based on the clinical context, patient conditions, and treatment plans. This reduces the chances of miscommunication and ensures that patients follow post-care instructions properly.
Key Benefits:
- Generates personalized instructions for patients
- Reduces the risk of patient non-compliance
- Improves patient outcomes by ensuring accurate post-care follow-up
11. Speech Recognition
Speech recognition technology, powered by NLP, allows healthcare providers to dictate notes and patient histories directly into their electronic health records. This technology saves time compared to manual data entry and reduces transcription errors. It’s particularly useful in fast-paced environments where clinicians need to document large volumes of information quickly.
Key Benefits:
- Speeds up documentation processes
- Reduces transcription errors
- Enhances clinician productivity and focus on patient care
12. Computer-Assisted Coding (CAC)
CAC systems use NLP to automate the medical coding process by analyzing clinical texts and automatically assigning appropriate codes. This technology significantly reduces the time healthcare providers spend on coding while improving accuracy, which is crucial for insurance claims and billing.
Key Benefits:
- Speeds up the coding process
- Improves the accuracy of medical codes
- Reduces administrative burden and costs for healthcare providers
13. Review Management and Sentiment Analysis
Beyond patient feedback, NLP tools can also analyze online reviews, comments, and social media posts to gauge public sentiment toward healthcare providers or institutions. This helps hospitals and clinics improve their reputation and address issues that might otherwise go unnoticed.
Key Benefits:
- Analyzes patient sentiment across multiple platforms
- Improves healthcare institution reputation management
- Identifies potential service improvements based on public feedback
14. Dictation and EMR Implications
Dictation is a common method for clinicians to record patient information, but without NLP, it can lead to errors or inconsistencies. By integrating NLP with dictation systems, clinicians can have their spoken words transcribed into structured, readable text that can be directly entered into EMRs, improving efficiency and reducing documentation errors.
Key Benefits:
- Enhances dictation accuracy
- Streamlines documentation for clinical staff
- Reduces documentation errors in EMRs
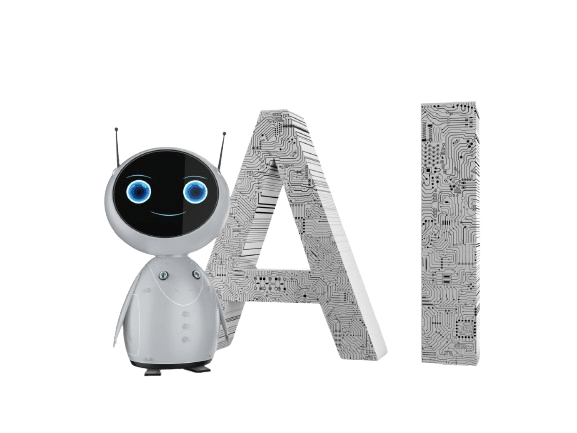
NLP Healthcare Models You Should Know About
Healthcare data is often messy and unstructured, making it difficult to extract meaningful insights. NLP models are designed to solve this problem by processing and analyzing medical text, from patient records to research articles. These models help streamline workflows, ensure privacy, and improve clinical decision-making.
Here are some key NLP models in healthcare that are driving this transformation:
1. Health Relation Extraction Model (HRE)
This model extracts relationships between medical entities, such as symptoms, diseases, and treatments, to build structured knowledge from unstructured text.
2. Health Assertion Detection (HASD)
HASD models identify whether a specific health-related statement is true, false, or uncertain. For example, “Patient is allergic to penicillin” might be marked as a true assertion.
3. Clinical Deidentification Model
This model helps remove personally identifiable information (PII) from patient records to ensure privacy and comply with regulations like HIPAA (Health Insurance Portability and Accountability Act).
4. Healthcare Named Entity Recognition (HNER)
HNER models identify medical terms such as drug names, diseases, and procedures in text, helping researchers and clinicians find specific information more efficiently.
5. Optical Character Recognition (OCR)
OCR technology enables healthcare providers to convert paper-based documents (e.g., prescriptions, medical records) into editable, searchable formats.
Challenges and Limitations of NLP in Healthcare
While NLP holds immense potential in healthcare, there are several limitations and challenges to its widespread integration:
1. Data Limitations
Healthcare data is often fragmented, siloed, or incomplete. For NLP systems to work effectively, they require clean, comprehensive, and high-quality data. This is often a barrier to successful implementation.
2. Linguistic Complexity
Medical language is complex, with its own set of terms, abbreviations, and jargon. NLP models must be trained to understand this specialized vocabulary, which can be a difficult and time-consuming task.
3. Regulatory Compliance Issues
Healthcare is highly regulated, especially regarding patient privacy. NLP systems must comply with laws like HIPAA in the U.S. Any NLP solution must ensure that sensitive patient information is protected at all times.
4. System Integration
Healthcare systems are diverse and often rely on outdated technology. Integrating NLP tools with existing systems, such as Electronic Health Records (EHRs) or legacy software, can be challenging and require significant resources.
How to Evaluate Readiness of Your Healthcare NLP Solution
Statista pointed out some of the most important factors or requirements that need to be considered when evaluating the readiness of machine learning, NLP or computer vision solution.
- State-of-the-art accuracy
- Implements healthcare specific models and algorithms
- Ability to train your own models
- Scalability to large datasets
- Healthcare-specific pre-trained models
- Production ready codebase
- Implements state-of-the-art techniques
- Fits with existing software stack
- No data sharing with software vendor
- Open-source, or open core software
Wrapping Up
From improving clinical trial management to enhancing doctors’ decision-making, NLP is revolutionizing how healthcare operates. However, challenges such as data quality, regulatory compliance, and system integration must be addressed for NLP’s full potential to be realized. With careful implementation and continuous innovation, NLP could soon become an indispensable tool for healthcare professionals worldwide.